Abstract
Neurotechnologies combine engineering methods and neuroscientific knowledge to design devices that interface the brain with the outside world. Since the early 2000s, inspiring and encouraging neurotechnology examples have been the subject of high-profile scientific articles and made headlines in popular media. However, although neurotechnologies have the potential to improve people’s lives in ways that cannot be achieved by other solutions such as pharmaceuticals, only a few of them have established themselves as clinical solutions. In this Review, we provide a systematic, state-of-the-art assessment of the opportunities and shortcomings of neurotechnology’s engineering and scientific components, and highlight the requirements to overcome translational barriers. Finally, we present a comprehensive framework to aid the clinical and commercial translation of neurotechnologies.
Key points
-
Neurotechnologies provide new diagnostic and treatment solutions in ways that cannot be achieved by other strategies such as pharmaceuticals, therefore potentially addressing the unmet needs of a large number of patients.
-
Despite important scientific and technical breakthroughs, as well as impressive demonstrations, only a few neurotechnologies have firmly established themselves as clinical solutions.
-
Developing successful neurotechnologies requires addressing technical, clinical and commercial requirements to overcome translational barriers.
-
Rigorously assessing and validating potential benefits, incorporating and advancing neuroscientific knowledge, optimizing technology innovation, and focusing on the user and on the right problem can assist the development of new solutions.
This is a preview of subscription content, access via your institution
Access options
Subscribe to this journal
Receive 12 digital issues and online access to articles
$99.00 per year
only $8.25 per issue
Buy this article
- Purchase on Springer Link
- Instant access to full article PDF
Prices may be subject to local taxes which are calculated during checkout
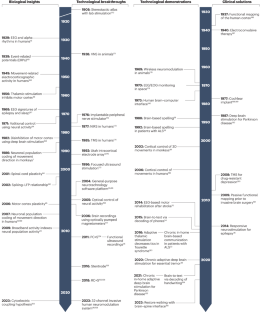
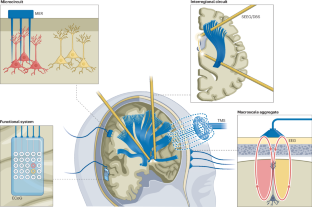
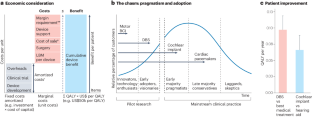
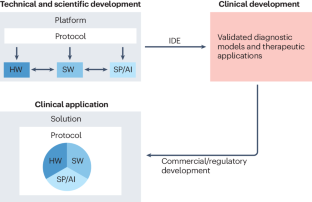
Similar content being viewed by others
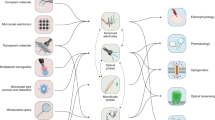
Recent advances in neurotechnologies with broad potential for neuroscience research
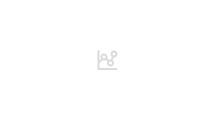
Navigating the FDA regulatory landscape
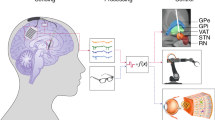
Precision electronic medicine in the brain
References
-
Wolpaw, J. & Wolpaw, E. (eds.) Brain–Computer Interfaces: Principles and Practice (Oxford Univ. Press, 2012).
-
Stieglitz, T. Of man and mice: translational research in neurotechnology. Neuron 105, 12–15 (2020).
-
Borton, D. A., Dawes, H. E., Worrell, G. A., Starr, P. A. & Denison, T. J. Developing collaborative platforms to advance neurotechnology and its translation. Neuron 108, 286–301 (2020).
-
Famm, K., Litt, B., Tracey, K. J., Boyden, E. S. & Slaoui, M. Drug discovery: a jump-start for electroceuticals. Nature 496, 159–161 (2013).
-
Mountcastle, V. B. Modality and topographic properties of single neurons of cat’s somatic sensory cortex. J. Neurophysiol. 20, 408–434 (1957).
-
Hubel, D. H. & Wiesel, T. N. Receptive fields, binocular interaction and functional architecture in the cat’s visual cortex. J. Physiol. 160, 106 (1962).
-
Georgopoulos, A. P., Schwartz, A. B. & Kettner, R. E. Neuronal population coding of movement direction. Science 233, 1416–1419 (1986).
-
Jun, J. J. et al. Fully integrated silicon probes for high-density recording of neural activity. Nature 551, 232–236 (2017).
-
Jones, K. E., Campbell, P. K. & Normann, R. A. A glass/silicon composite intracortical electrode array. Ann. Biomed. Eng. 20, 423–437 (1992).
-
Shain, W. et al. Controlling cellular reactive responses around neural prosthetic devices using peripheral and local intervention strategies. IEEE Trans. Neural Syst. Rehabil. Eng. 11, 186–188 (2003).
-
Ritaccio, A. L., Brunner, P. & Schalk, G. Electrical stimulation mapping of the brain: basic principles and emerging alternatives. J. Clin. Neurophysiol. 35, 86 (2018).
-
Kubanek, J., Miller, K. J., Ojemann, J. G., Wolpaw, J. R. & Schalk, G. Decoding flexion of individual fingers using electrocorticographic signals in humans. J. Neural Eng. 6, 066001 (2009).
-
Miller, K. et al. Spectral changes in cortical surface potentials during motor movement. J. Neurosci. 27, 2424–2432 (2007).
-
Schalk, G. et al. Decoding two-dimensional movement trajectories using electrocorticographic signals in humans. J. Neural Eng. 4, 264–275 (2007).
-
Berger, H. Über das Electroenkephalogramm des Menschen. Arch. Psychiat. Nervenkr. 87, 527–570 (1929).
-
Buzsáki, G. & Draguhn, A. Neuronal oscillations in cortical networks. Science 304, 1926–1929 (2004).
-
Haegens, S., Nácher, V., Luna, R., Romo, R. & Jensen, O. α-Oscillations in the monkey sensorimotor network influence discrimination performance by rhythmical inhibition of neuronal spiking. Proc. Natl Acad. Sci. USA 108, 19377–19382 (2011).
-
Coon, W. G. et al. Oscillatory phase modulates the timing of neuronal activations and resulting behavior. NeuroImage 133, 294–301 (2016).
-
Moheimanian, L., Paraskevopoulou, S. E., Adamek, M., Schalk, G. & Brunner, P. Modulation in cortical excitability disrupts information transfer in perceptual-level stimulus processing. NeuroImage 243, 118498 (2021).
-
Miller, K. J., Sorensen, L. B., Ojemann, J. G. & den Nijs, M. Power-law scaling in the brain surface electric potential. PLoS Comput. Biol. 5, e1000609 (2009).
-
Manning, J. R., Jacobs, J., Fried, I. & Kahana, M. J. Broadband shifts in local field potential power spectra are correlated with single-neuron spiking in humans. J. Neurosci. 29, 13613–13620 (2009).
-
Ray, S. & Maunsell, J. Different origins of gamma rhythm and high-gamma activity in macaque visual cortex. PLoS Biol. 9, e1000610 (2011).
-
Whittingstall, K. & Logothetis, N. K. Frequency-band coupling in surface EEG reflects spiking activity in monkey visual cortex. Neuron 64, 281–289 (2009).
-
Gardner, A. B., Worrell, G. A., Marsh, E., Dlugos, D. & Litt, B. Human and automated detection of high-frequency oscillations in clinical intracranial EEG recordings. Clin. Neurophysiol. 118, 1134–1143 (2007).
-
Crowther, L. J. et al. A quantitative method for evaluating cortical responses to electrical stimulation. J. Neurosci. Methods 311, 67–75 (2019).
-
Miller, K. J. et al. Canonical response parameterization: quantifying the structure of responses to single-pulse intracranial electrical brain stimulation. PLoS Comput. Biol. 19(5), e1011105 (2023).
-
Butson, C. R. & McIntyre, C. C. Current steering to control the volume of tissue activated during deep brain stimulation. Brain Stimul. 1, 7–15 (2008).
-
Blakely, T., Miller, K. J., Zanos, S. P., Rao, R. P. & Ojemann, J. G. Robust, long-term control of an electrocorticographic brain–computer interface with fixed parameters. Neurosurg. Focus. 27, E13 (2009).
-
Chao, Z. C., Nagasaka, Y. & Fujii, N. Long-term asynchronous decoding of arm motion using electrocorticographic signals in monkeys. Front. Neuroeng. https://ift.tt/9pmb2Dk (2010).
-
Schalk, G. Can electrocorticography (ECoG) support robust and powerful brain-computer interfaces? Front. Neuroeng. 3, 9 (2010).
-
Leuthardt, E. C., Schalk, G., Wolpaw, J. R., Ojemann, J. G. & Moran, D. W. A brain-computer interface using electrocorticographic signals in humans. J. Neural Eng. 1, 63–71 (2004).
-
Schalk, G. et al. Two-dimensional movement control using electrocorticographic signals in humans. J. Neural Eng. 5, 75 (2008).
-
Vansteensel, M. J. et al. Fully implanted brain–computer interface in a locked-in patient with ALS. N. Engl. J. Med. 375, 2060–2066 (2016).
-
Herff, C. et al. Brain-to-text: decoding spoken phrases from phone representations in the brain. Front. Neurosci. 9, 217 (2015).
-
Moses, D. A. et al. Neuroprosthesis for decoding speech in a paralyzed person with anarthria. N. Engl. J. Med. 385, 217–227 (2021).
-
Heck, C. N. et al. Two-year seizure reduction in adults with medically intractable partial onset epilepsy treated with responsive neurostimulation: final results of the RNS system pivotal trial. Epilepsia 55, 432–441 (2014).
-
Edwardson, M., Lucas, T., Carey, J. & Fetz, E. New modalities of brain stimulation for stroke rehabilitation. Exp. Brain Res. 224, 335–358 (2013).
-
de Hemptinne, C. et al. Exaggerated phase-amplitude coupling in the primary motor cortex in Parkinson disease. Proc. Natl Acad. Sci. USA 110, 4780–4785 (2013).
-
Opri, E. et al. Chronic embedded cortico-thalamic closed-loop deep brain stimulation for the treatment of essential tremor. Sci. Transl. Med. 12, eaay7680 (2020).
-
Scangos, K. W. et al. Closed-loop neuromodulation in an individual with treatment-resistant depression. Nat. Med. 27, 1696–1700 (2021).
-
Kubanek, J. Neuromodulation with transcranial focused ultrasound. Neurosurg. Focus 44, E14 (2018).
-
Philip, N. S. & Arulpragasam, A. R. Reaching for the unreachable: low intensity focused ultrasound for non-invasive deep brain stimulation. Neuropsychopharmacology 48, 251–252 (2022).
-
Mirzakhalili, E., Barra, B., Capogrosso, M. & Lempka, S. F. Biophysics of temporal interference stimulation. Cell Syst. 11, 557–572 (2020).
-
Acerbo, E. et al. Focal non-invasive deep-brain stimulation with temporal interference for the suppression of epileptic biomarkers. Front. Neurosci. 16, 945221 (2022).
-
Macé, E. et al. Functional ultrasound imaging of the brain. Nat. Methods 8, 662–664 (2011).
-
Li, C.-L. The inhibitory effect of stimulation of a thalamic nucleus on neuronal activity in the motor cortex. J. Physiol. 133, 40–53 (1956).
-
Pfurtscheller, G. et al. The hybrid BCI. Front. Neurosci. https://ift.tt/DZsE8wh (2010).
-
Müller-Putz, G. et al. Towards noninvasive hybrid brain–computer interfaces: framework, practice, clinical application, and beyond. Proc. IEEE 103, 926–943 (2015).
-
Ross, S. D. et al. Systematic review of the literature regarding the diagnosis of sleep apnea. Evid. Rep. Technol. Assess. https://ift.tt/AbiDEHB (1998).
-
Smith, S. J. EEG in the diagnosis, classification, and management of patients with epilepsy. J. Neurol. Neurosurg. Psychiatry 76, ii2–ii7 (2005).
-
Blackhart, G. C., Minnix, J. A. & Kline, J. P. Can EEG asymmetry patterns predict future development of anxiety and depression? A preliminary study. Biol. Psychol. 72, 46–50 (2006).
-
Thibodeau, R., Jorgensen, R. S. & Kim, S. Depression, anxiety, and resting frontal EEG asymmetry: a meta-analytic review. J. Abnorm. Psychol. 115, 715 (2006).
-
Mohan, Y., Chee, S. S., Xin, D. K. P. & Foong, L. P. Artificial neural network for classification of depressive and normal in EEG. In 2016 IEEE EMBS Conference on Biomedical Engineering and Sciences (IECBES), 286–290 (IEEE, 2016).
-
de Aguiar Neto, F. S. & Rosa, J. L. G. Depression biomarkers using non-invasive EEG: a review. Neurosci. Biobehav. Rev. 105, 83–93 (2019).
-
Jiang, C., Li, Y., Tang, Y. & Guan, C. Enhancing EEG-based classification of depression patients using spatial information. IEEE Trans. Neural Syst. Rehabil. Eng. 29, 566–575 (2021).
-
Nuwer, M. R., Hovda, D. A., Schrader, L. M. & Vespa, P. M. Routine and quantitative EEG in mild traumatic brain injury. Clin. Neurophysiol. 116, 2001–2025 (2005).
-
Doan, D. N. T. et al. Predicting dementia with prefrontal electroencephalography and event-related potential. Front. Aging Neurosci. 13, 659817 (2021).
-
Farwell, L. A. & Donchin, E. Talking off the top of your head: toward a mental prosthesis utilizing event-related brain potentials. Electroencephalogr. Clin. Neurophysiol. 70, 510–523 (1988).
-
Sutter, E. E. The brain response interface: communication through visually-induced electrical brain responses. J. Microcomput. Appl. 15, 31–45 (1992).
-
Pfurtscheller, G., Flotzinger, D. & Kalcher, J. Brain–computer interface — a new communication device for handicapped persons. J. Microcomput. Appl. 16, 293–299 (1993).
-
Birbaumer, N. et al. A spelling device for the paralysed. Nature 398, 297–298 (1999).
-
Wolpaw, J. R., Birbaumer, N., McFarland, D. J., Pfurtscheller, G. & Vaughan, T. M. Brain–computer interfaces for communication and control. Clin. Neurophysiol. 113, 767–791 (2002).
-
Kübler, A. et al. Patients with ALS can use sensorimotor rhythms to operate a brain–computer interface. Neurology 64, 1775–1777 (2005).
-
Sellers, E. W. & Donchin, E. A P300-based brain–computer interface: initial tests by ALS patients. Clin. Neurophysiol. 117, 538–548 (2006).
-
Müller-Putz, G. R., Scherer, R., Brauneis, C. & Pfurtscheller, G. Steady-state visual evoked potential (SSVEP)-based communication: impact of harmonic frequency components. J. Neural Eng. 2, 123 (2005).
-
Wolpaw, J. R. in Handbook of Clinical Neurology Vol. 110, 67–74 (Elsevier, 2013).
-
Chen, X. et al. High-speed spelling with a noninvasive brain–computer interface. Proc. Natl Acad. Sci. USA 112, E6058–E6067 (2015).
-
Soekadar, S. et al. Hybrid EEG/EOG-based brain/neural hand exoskeleton restores fully independent daily living activities after quadriplegia. Sci. Robot. 1, eaag3296 (2016).
-
Chaudhary, U. et al. Spelling interface using intracortical signals in a completely locked-in patient enabled via auditory neurofeedback training. Nat. Commun. 13, 1236 (2022).
-
Ang, K. K. et al. Brain–computer interface-based robotic end effector system for wrist and hand rehabilitation: results of a three-armed randomized controlled trial for chronic stroke. Front. Neuroeng. 7, 30 (2014).
-
Soekadar, S. R., Birbaumer, N., Slutzky, M. W. & Cohen, L. G. Brain–machine interfaces in neurorehabilitation of stroke. Neurobiol. Dis. 83, 172–179 (2015).
-
Bundy, D. T. et al. Contralesional brain–computer interface control of a powered exoskeleton for motor recovery in chronic stroke survivors. Stroke 48, 1908–1915 (2017).
-
Cervera, M. A. et al. Brain-computer interfaces for post-stroke motor rehabilitation: a meta-analysis. Ann. Clin. Transl. Neurol. 5, 651–663 (2018).
-
Musso, M. et al. Aphasia recovery by language training using a brain–computer interface: a proof-of-concept study. Brain Commun. 4, fcac008 (2022).
-
Donati, A. R. et al. Long-term training with a brain–machine interface-based gait protocol induces partial neurological recovery in paraplegic patients. Sci. Rep. 6, 30383 (2016).
-
Sonmez, A. I. et al. Accelerated TMS for depression: a systematic review and meta-analysis. Psychiatry Res. 273, 770–781 (2019).
-
Trevizol, A. P. et al. Transcranial magnetic stimulation for obsessive-compulsive disorder: an updated systematic review and meta-analysis. J. ECT 32, 262–266 (2016).
-
Cole, J. C., Bernacki, C. G., Helmer, A., Pinninti, N. & O’reardon, J. P. Efficacy of transcranial magnetic stimulation (TMS) in the treatment of schizophrenia: a review of the literature to date. Innov. Clin. Neurosci. 12, 12 (2015).
-
Cirillo, G. et al. Neurobiological after-effects of non-invasive brain stimulation. Brain Stimul. 10, 1–18 (2017).
-
Mantegazza, M., Curia, G., Biagini, G., Ragsdale, D. S. & Avoli, M. Voltage-gated sodium channels as therapeutic targets in epilepsy and other neurological disorders. Lancet Neurol. 9, 413–424 (2010).
-
Adaikkan, C. et al. Gamma entrainment binds higher-order brain regions and offers neuroprotection. Neuron 102, 929–943 (2019).
-
Soula, M. et al. Forty-hertz light stimulation does not entrain native gamma oscillations in Alzheimer’s disease model mice. Nat. Neurosci. 26, 570–578 (2023).
-
Nasr, K. et al. Breaking the boundaries of interacting with the human brain using adaptive closed-loop stimulation. Prog. Neurobiol. 226, 102311 (2022).
-
Wolpaw, J. R. & Tennissen, A. M. Activity-dependent spinal cord plasticity in health and disease. Annu. Rev. Neurosci. 24, 807–843 (2001).
-
Jackson, A., Mavoori, J. & Fetz, E. E. Long-term motor cortex plasticity induced by an electronic neural implant. Nature 444, 56–60 (2006).
-
Haslacher, D. et al. In vivo phase-dependent enhancement and suppression of human brain oscillations by transcranial alternating current stimulation (tACS). NeuroImage 275, 120187 (2023).
-
Ngo, H.-V. V., Martinetz, T., Born, J. & Mölle, M. Auditory closed-loop stimulation of the sleep slow oscillation enhances memory. Neuron 78, 545–553 (2013).
-
Santostasi, G. et al. Phase-locked loop for precisely timed acoustic stimulation during sleep. J. Neurosci. Methods 259, 101–114 (2016).
-
Garcia-Molina, G. et al. Closed-loop system to enhance slow-wave activity. J. Neural Eng. 15, 066018 (2018).
-
Lustenberger, C. et al. Auditory deep sleep stimulation in older adults at home: a randomized crossover trial. Commun. Med. 2, 30 (2022).
-
Ehrlich, S. K., Agres, K. R., Guan, C. & Cheng, G. A closed-loop, music-based brain–computer interface for emotion mediation. PLoS ONE 14, e0213516 (2019).
-
Clausen, J. et al. Help, hope, and hype: ethical dimensions of neuroprosthetics. Science 356, 1338–1339 (2017).
-
Soekadar, S., Chandler, J., Ienca, M. & Bublitz, C. On the verge of the hybrid mind. Morals Mach. 1, 30–43 (2021).
-
Fetz, E. E. & Finocchio, D. V. Operant conditioning of specific patterns of neural and muscular activity. Science 174, 431–435 (1971).
-
Schalk, G., McFarland, D., Hinterberger, T., Birbaumer, N. & Wolpaw, J. BCI2000: a general-purpose brain–computer interface (BCI) system. IEEE Trans. Biomed. Eng. 51, 1034–1043 (2004).
-
Schalk, G. & Mellinger, J. A Practical Guide to Brain–Computer Interfacing with BCI2000 (Springer, 2010).
-
Renard, Y. et al. OpenViBE: an open-source software platform to design, test, and use brain–computer interfaces in real and virtual environments. Presence 19, 35–53 (2010).
-
Brunner, P. & Schalk, G. in Brain–Computer Interfaces Handbook: Technological and Theoretical Advances (eds Nam, C. S. et al.) 323–336 (CRC, 2018).
-
Schalk, G. et al. Toward a fully implantable ecosystem for adaptive neuromodulation in humans: preliminary experience with the CorTec BrainInterchange device in a canine model. Front. Neurosci. 16, 932782 (2022).
-
Dornhege, G. et al. Combined optimization of spatial and temporal filters for improving brain-computer interfacing. IEEE Trans. Biomed. Eng. 53, 2274–2281 (2006).
-
McFarland, D., Anderson, C., Muller, K.-R., Schlogl, A. & Krusienski, D. BCI meeting 2005 — workshop on BCI signal processing: feature extraction and translation. IEEE Trans. Neural Syst. Rehabil. Eng. 14, 135–138 (2006).
-
Gilja, V. et al. Clinical translation of a high-performance neural prosthesis. Nat. Med. 21, 1142–1145 (2015).
-
Ang, K. K., Chin, Z. Y., Zhang, H. & Guan, C. Filter bank common spatial pattern (FBCSP) in brain–computer interface. In 2008 IEEE International Joint Conference on Neural Networks (IEEE World Congress on Computational Intelligence) 2390–2397 (IEEE, 2008).
-
Lotte, F. & Guan, C. Regularizing common spatial patterns to improve BCI designs: unified theory and new algorithms. IEEE Trans. Biomed. Eng. 58, 355–362 (2011).
-
Wei, Q., Wang, Y., Gao, X. & Gao, S. Amplitude and phase coupling measures for feature extraction in an EEG-based brain–computer interface. J. Neural Eng. 4, 120 (2007).
-
Lotte, F. et al. A review of classification algorithms for EEG-based brain–computer interfaces: a 10 year update. J. Neural Eng. 15, 031005 (2018).
-
Aru, J. et al. Untangling cross-frequency coupling in neuroscience. Curr. Opin. Neurobiol. 31, 51–61 (2015).
-
Schalk, G. A general framework for dynamic cortical function: the function-through-biased-oscillations (FBO) hypothesis. Front. Hum. Neurosci. 9, 352 (2015).
-
Krusienski, D. J., Schalk, G., McFarland, D. J. & Wolpaw, J. R. A mu-rhythm matched filter for continuous control of a brain–computer interface. IEEE Trans. Biomed. Eng. 54, 273–280 (2007).
-
Schalk, G., Marple, J., Knight, R. T. & Coon, W. G. Instantaneous voltage as an alternative to power- and phase-based interpretation of oscillatory brain activity. Neuroimage 157, 545–554 (2017).
-
Homer, M., Nurmikko, A., Donoghue, J. & Hochberg, L. Sensors and decoding for intracortical brain computer interfaces. Annu. Rev. Biomed. Eng. 15, 383–405 (2013).
-
Zhang, K., Robinson, N., Lee, S.-W. & Guan, C. Adaptive transfer learning for EEG motor imagery classification with deep convolutional neural network. Neural Netw. 136, 1–10 (2021).
-
Kwon, O.-Y., Lee, M.-H., Guan, C. & Lee, S.-W. Subject-independent brain–computer interfaces based on deep convolutional neural networks. IEEE Trans. Neural Netw. Learn. Syst. 31, 3839–3852 (2020).
-
Delorme, A., Sejnowski, T. & Makeig, S. Enhanced detection of artifacts in EEG data using higher-order statistics and independent component analysis. NeuroImage 34, 1443–1449 (2007).
-
Islam, M. K., Rastegarnia, A. & Yang, Z. Methods for artifact detection and removal from scalp EEG: a review. Neurophysiol. Clin. 46, 287–305 (2016).
-
Chang, C.-Y., Hsu, S.-H., Pion-Tonachini, L. & Jung, T.-P. Evaluation of artifact subspace reconstruction for automatic artifact components removal in multi-channel EEG recordings. IEEE Trans. Biomed. Eng. 67, 1114–1121 (2020).
-
Winkler, I., Haufe, S. & Tangermann, M. Automatic classification of artifactual ICA-components for artifact removal in EEG signals. Behav. Brain Funct. 7, 1–15 (2011).
-
Mullen, T. R. et al. Real-time neuroimaging and cognitive monitoring using wearable dry EEG. IEEE Trans. Biomed. Eng. 62, 2553–2567 (2015).
-
Blum, S., Jacobsen, N. S., Bleichner, M. G. & Debener, S. A Riemannian modification of artifact subspace reconstruction for EEG artifact handling. Front. Hum. Neurosci. 13, 141 (2019).
-
Schreyögg, J., Bäumler, M. & Busse, R. Balancing adoption and affordability of medical devices in Europe. Health Policy 92, 218–224 (2009).
-
Hall, B. H. & Khan, B. Adoption of New Technology. https://ift.tt/eQBitLx (2003).
-
Kilgore, K. L. et al. An implanted upper-extremity neuroprosthesis using myoelectric control. J. Hand Surg. 33, 539–550 (2008).
-
Haugland, M. et al. A preliminary non-randomised study to evaluate the safety and performance of the ActiGait implanted drop-foot stimulator in established hemiplegia. In Getting FES into Clinical Practice, Proc. IFESS-FESnet 2004 (eds Wood, D. & Taylor, J.) 153–155 (2004).
-
Luo, Y. H.-L. & Da Cruz, L. The Argus® II retinal prosthesis system. Prog. Retin. Eye Res. 50, 89–107 (2016).
-
Bergstein, B. Paralyzed again. MIT Technol. Rev. (9 April 2015).
-
Strickland, E. & Harris, M. What happens when a bionic body part becomes obsolete? Blind people with Second Sight’s retinal implants found out. IEEE Spectr. 59, 24–31 (2022).
-
Drew, L. Abandoned: the human cost of neurotechnology failure. Nature https://ift.tt/AIliGXM (2022).
-
Kramer, D. B., Xu, S. & Kesselheim, A. S. How does medical device regulation perform in the United States and the European Union? A systematic review. PLoS Med. 9, e1001276 (2012).
-
Shiroiwa, T. et al. International survey on willingness-to-pay (WTP) for one additional QALY gained: what is the threshold of cost effectiveness? Heal. Econ. 19, 422–437 (2010).
-
Benabid, A.-L., Pollak, P., Louveau, A., Henry, S. & De Rougemont, J. Combined (thalamotomy and stimulation) stereotactic surgery of the VIM thalamic nucleus for bilateral Parkinson disease. Stereotact. Funct. Neurosurg. 50, 344–346 (1987).
-
Kestenbaum M, L. E. & Ford, B. Estimating the proportion of essential tremor and Parkinson’s disease patients undergoing deep brain stimulation surgery: five-year data from Columbia University Medical Center (2009–2014). Mov. Disord. Clin. Pract. 4, 384–378 (2015).
-
Morgante, L. et al. How many Parkinsonian patients are suitable candidates for deep brain stimulation of subthalamic nucleus? Results of a questionnaire. Park. Relat. Disord. 13, 528–531 (2007).
-
Nassiri, A. M., Sorkin, D. L. & Carlson, M. L. Current estimates of cochlear implant utilization in the United States. Otol. Neurotol. 43, e558–e562 (2022).
-
Smilowska, K. et al. Cost-effectiveness of device-aided therapies in Parkinson’s disease: a structured review. J. Park. Dis. 11, 475–489 (2021).
-
Cheng, L.-J., Soon, S. S., Wu, D. B.-C., Ju, H. & Ng, K. Cost-effectiveness analysis of bilateral cochlear implants for children with severe-to-profound sensorineural hearing loss in both ears in Singapore. PLoS ONE 14, e0220439 (2019).
-
Montes, F. et al. Cochlear implants versus hearing aids in a middle-income country: costs, productivity, and quality of life. Otol. Neurotol. 38, e26–e33 (2017).
-
Qiu, J. et al. Cost-effectiveness of pediatric cochlear implantation in rural China. Otol. Neurotol. 38, e75 (2017).
-
Tolstoy, L. Anna Karenina (The Russian Messenger, 1878).
-
Diamond, J. Guns, Germs, and Steel: The Fates of Human Societies 20th anniversary edn (W. W. Norton & Company, 2017).
-
Brooks, F. P. & Bullet, N. S. Essence and accidents of software engineering. IEEE Comput. 20, 10–19 (1987).
-
Fetz, E. E. Real-time control of a robotic arm by neuronal ensembles. Nat. Neurosci. 2, 583–584 (1999).
-
Chapin, J. K. Neural prosthetic devices for quadriplegia. Curr. Opin. Neurol. 13, 671–675 (2000).
-
Nicolelis, M. A. Actions from thoughts. Nature 409, 403–407 (2001).
-
Donoghue, J. P. Connecting cortex to machines: recent advances in brain interfaces. Nat. Neurosci. 5, 1085–1088 (2002).
-
Lyle, R. C. A performance test for assessment of upper limb function in physical rehabilitation treatment and research. Int. J. Rehabil. Res. 4, 483–492 (1981).
-
Buhmann, C. et al. Adverse events in deep brain stimulation: a retrospective long-term analysis of neurological, psychiatric and other occurrences. PLoS ONE 12, e0178984 (2017).
-
Follett, K. A. et al. Pallidal versus subthalamic deep-brain stimulation for Parkinson’s disease. N. Engl. J. Med. 362, 2077–2091 (2010).
-
Figee, M. et al. Deep brain stimulation for depression. Neurotherapeutics 19, 1229–1245 (2022).
-
Levy, R. M. The need for mechanism-based medicine in neuromodulation. Neuromodulation 15, 273–279 (2012).
-
Abou-Al-Shaar, H., Brock, A. A., Kundu, B., Englot, D. J. & Rolston, J. D. Increased nationwide use of stereoencephalography for intracranial epilepsy electroencephalography recordings. J. Clin. Neurosci. 53, 132–134 (2018).
-
Li, G. et al. Optimal referencing for stereo-electroencephalographic (SEEG) recordings. NeuroImage 183, 327–335 (2018).
-
Coon, W. & Schalk, G. A method to establish the spatiotemporal evolution of task-related cortical activity from electrocorticographic signals in single trials. J. Neurosci. Methods 271, 76–85 (2016).
-
Morrell, M. Responsive cortical stimulation for the treatment of medically intractable partial epilepsy. Neurology 77, 1295–1304 (2011).
-
Rouse, A. G. et al. A chronic generalized bi-directional brain–machine interface. J. Neural Eng. 8, 036018 (2011).
-
Gilron, R. et al. Long-term wireless streaming of neural recordings for circuit discovery and adaptive stimulation in individuals with Parkinson’s disease. Nat. Biotechnol. 39, 1078–1085 (2021).
-
Stanslaski, S. et al. A chronically implantable neural coprocessor for investigating the treatment of neurological disorders. IEEE Trans. Biomed. Circuits Syst. 12, 1230–1245 (2018).
-
Jimenez-Shahed, J. Device profile of the percept PC deep-brain stimulation system for the treatment of Parkinson’s disease and related disorders. Expert. Rev. Med. Devices 18, 319–332 (2021).
-
Kohler, F. et al. Closed-loop interaction with the cerebral cortex: a review of wireless implant technology. Brain Comput. Interfaces 4, 146–154 (2017).
-
Oxley, T. J. et al. Minimally invasive endovascular stent-electrode array for high-fidelity, chronic recordings of cortical neural activity. Nat. Biotechnol. 34, 320–327 (2016).
-
Musk, E. et al. An integrated brain–machine interface platform with thousands of channels. J. Med. Internet Res. 21, e16194 (2019).
-
Peckham, P. H. & Kilgore, K. L. Challenges and opportunities in restoring function after paralysis. IEEE Trans. Biomed. Eng. 60, 602–609 (2013).
-
Chen, X. Y. & Wolpaw, J. R. Operant conditioning of H-reflex in freely moving rats. J. Neurophysiol. 73, 411–415 (1995).
-
Thompson, A. K. & Wolpaw, J. R. H-reflex conditioning during locomotion in people with spinal cord injury. J. Physiol. 599, 2453–2469 (2019).
-
Sebastián-Romagosa, M. et al. Brain computer interface treatment for motor rehabilitation of upper extremity of stroke patients — a feasibility study. Front. Neurosci. 14, 591435 (2020).
-
Davis, P. A. Effects of acoustic stimuli on the waking human brain. J. Neurophysiol. 2, 494–499 (1939).
-
Jasper, H. & Penfield, W. Electrocorticograms in man: effect of voluntary movement upon the electrical activity of the precentral gyrus. Arch. für Psychiatr. und Nervenkrankheiten 183, 163–174 (1949).
-
Gibbs, F. A., Davis, H. & Lennox, W. G. The electroencephalogram in epilepsy and in conditions of impaired consciousness. Am. J. EEG Technol. 8, 59–73 (1968).
-
Cooper, I. S., Upton, A. & Amin, I. Chronic cerebellar stimulation (CCS) and deep brain stimulation (DBS) in involuntary movement disorders. Appl. Neurophysiol. 45, 209–217 (1982).
-
Pistohl, T., Ball, T., Schulze-Bonhage, A., Aertsen, A. & Mehring, C. Prediction of arm movement trajectories from ECoG-recordings in humans. J. Neurosci. Methods 167, 105–114 (2008).
-
Horsley, V. & Clarke, R. H. The structure and functions of the cerebellum examined by a new method. Brain 31, 45–124 (1908).
-
Bailey, P. & Bremer, F. A sensory cortical representation of the vagus nerve: with a note on the effects of low blood pressure on the cortical electrogram. J. Neurophysiol. 1, 405–412 (1938).
-
Laitinen, L. Placement of electrodes in transcutaneous stimulation for chronic pain. Neurochirurgie 22, 517–526 (1976).
-
Jöbsis, F. F. Noninvasive, infrared monitoring of cerebral and myocardial oxygen sufficiency and circulatory parameters. Science 198, 1264–1267 (1977).
-
Barker, A. T., Jalinous, R. & Freeston, I. L. Non-invasive magnetic stimulation of human motor cortex. Lancet 325, 1106–1107 (1985).
-
Gavrilov, L., Tsirulnikov, E. & Davies, I. a. I. Application of focused ultrasound for the stimulation of neural structures. Ultrasound Med. Biol. 22, 179–192 (1996).
-
Maynard, E. M., Nordhausen, C. T. & Normann, R. A. The Utah intracortical electrode array: a recording structure for potential brain–computer interfaces. Electroencephalogr. Clin. Neurophysiol. 102, 228–239 (1997).
-
Xia, H., Ben-Amar Baranga, A., Hoffman, D. & Romalis, M. Magnetoencephalography with an atomic magnetometer. Appl. Phys. Lett. 89, 211104 (2006).
-
Delgado, J. M., Bradley, R. J., Johnston, V. S., Weiss, G. & Wallace, J. D. Implantation of Multilead Electrode Assemblies and Radio Stimulation of the Brain in Chimpanzees. Technical Report (Yale Univ. Department of Psychiatry, 1969).
-
Frost, J. Jr Development of a Prototype Onboard EEG Analysis System. Technical Report CR 108508 (NASA, 1970).
-
Vidal, J. J. Toward direct brain–computer communication. Annu. Rev. Biophys. Bioeng. 2, 157–180 (1973).
-
Taylor, D. M., Tillery, S. I. & Schwartz, A. B. Direct cortical control of 3D neuroprosthetic devices. Science 296, 1829–1832 (2002).
-
Hochberg, L. R. et al. Neuronal ensemble control of prosthetic devices by a human with tetraplegia. Nature 442, 164–171 (2006).
-
Rossi, P. J. et al. Scheduled, intermittent stimulation of the thalamus reduces tics in Tourette syndrome. Park. Relat. Disord. 29, 35–41 (2016).
-
Willett, F. R., Avansino, D. T., Hochberg, L. R., Henderson, J. M. & Shenoy, K. V. High-performance brain-to-text communication via handwriting. Nature 593, 249–254 (2021).
-
Lorach, H. et al. Walking naturally after spinal cord injury using a brain–spine interface. Nature 618, 126–133 (2023).
-
Penfield, W. & Boldrey, E. Somatic motor and sensory representation in the cerebral cortex of man as studied by electrical stimulation. Brain 60, 389–443 (1937).
-
Cerletti, U. L’elettroshock. Riv. Sper. Freniat. Med. Leg. Alien. Ment. 64, 209–310 (1940).
-
Desoyer, I. & Hochmair, E. Implantable eight-channel stimulator for the deaf. In ESSCIRC’77: 3rd European Solid State Circuits Conference, 87–89 (IEEE, 1977).
-
Clark, G. M., Clark, J. C. & Furness, J. B. The evolving science of cochlear implants. JAMA 310, 1225–1226 (2013).
-
Pascual-Leone, A., Rubio, B., Pallardó, F. & Catalá, M. D. Rapid-rate transcranial magnetic stimulation of left dorsolateral prefrontal cortex in drug-resistant depression. Lancet 348, 233–237 (1996).
-
Brunner, P. et al. A practical procedure for real-time functional mapping of eloquent cortex using electrocorticographic signals in humans. Epilepsy Behav. 15, 278–286 (2009).
-
Pesaran, B., Pezaris, J. S., Sahani, M., Mitra, P. P. & Andersen, R. A. Temporal structure in neuronal activity during working memory in macaque parietal cortex. Nat. Neurosci. 5, 805–811 (2002).
-
Pinotsis, D. A., Fridman, G. & Miller, E. K. Cytoelectric coupling: electric fields sculpt neural activity and ‘tune’ the brain’s infrastructure. Prog. Neurobiol. 226, 102465 (2023).
-
Boyden, E. S., Zhang, F., Bamberg, E., Nagel, G. & Deisseroth, K. Millisecond-timescale, genetically targeted optical control of neural activity. Nat. Neurosci. 8, 1263–1268 (2005).
-
Moore, G. A. Crossing the Chasm: Marketing and Selling Disruptive Products to Mainstream Customers 3rd edn (Collins, 2014).
-
Kremen, V. et al. Integrating brain implants with local and distributed computing devices: a next generation epilepsy management system. IEEE J. Transl. Eng. Health Med. 6, 2500112 (2018).
-
Gunduz, A. et al. Adding wisdom to ‘smart’ bioelectronic systems: a design framework for physiologic control including practical examples. Bioelectron. Med. 2, 29–41 (2019).
-
Shirvalkar, P. et al. First-in-human prediction of chronic pain state using intracranial neural biomarkers. Nat. Neurosci. 26, 1090–1099 (2023).
-
Alagapan, S. et al. Cingulate dynamics track depression recovery with deep brain stimulation. Nature 622, 130–138 (2023).
-
Skarpaas, T. L., Jarosiewicz, B. & Morrell, M. J. Brain-responsive neurostimulation for epilepsy (RNS® system). Epilepsy Res. 153, 68–70 (2019).
-
Strollo, P. J. Jr et al. Upper-airway stimulation for obstructive sleep apnea. N. Engl. J. Med. 370, 139–149 (2014).
-
Ryu, S. I. & Shenoy, K. V. Human cortical prostheses: lost in translation? Neurosurg. Focus 27, E5 (2009).
-
Oxley, T. J. et al. Motor neuroprosthesis implanted with neurointerventional surgery improves capacity for activities of daily living tasks in severe paralysis: first in-human experience. J. Neurointerv. Surg. 13, 102–108 (2021).
-
Levy, R. M. et al. Epidural electrical stimulation for stroke rehabilitation: results of the prospective, multicenter, randomized, single-blinded Everest trial. Neurorehabil. Neural Repair. 30, 107–119 (2016).
-
Ezzyat, Y. et al. Closed-loop stimulation of temporal cortex rescues functional networks and improves memory. Nat. Commun. 9, 365 (2018).
-
Krauss, J. K. et al. Technology of deep brain stimulation: current status and future directions. Nat. Rev. Neurol. 17, 75–87 (2021).
-
Kostarelos, K., Vincent, M., Hebert, C. & Garrido, J. A. Graphene in the design and engineering of next-generation neural interfaces. Adv. Mater. 29, 1700909 (2017).
-
Peckham, P. H. et al. Efficacy of an implanted neuroprosthesis for restoring hand grasp in tetraplegia: a multicenter study. Arch. Phys. Med. Rehabil. 82, 1380–1388 (2001).
-
van der Aa, H., Alleman, E., Nene, A. & Snoek, G. Sacral anterior root stimulation for bladder control: clinical results. Arch. Physiol. Biochem. 107, 248–256 (1999).
-
da Cruz, L. et al. Five-year safety and performance results from the Argus II retinal prosthesis system clinical trial. Ophthalmology 123, 2248–2254 (2016).
-
Cook, M. J. et al. Prediction of seizure likelihood with a long-term, implanted seizure advisory system in patients with drug-resistant epilepsy: a first-in-man study. Lancet Neurol. 12, 563–571 (2013).
-
Gilbert, F., Ienca, M. & Cook, M. How I became myself after merging with a computer: does human–machine symbiosis raise human rights issues? Brain Stimul. 16, 783–789 (2023).
Acknowledgements
We acknowledge funding from the Tianqiao and Chrissy Chen Institute (G.S.), the RIE2020 AME Programmatic Fund in Singapore (A20G8b0102 (C.G.)), the NIH (U01-NS12861 (K.J.M.) and P41-EB018783 (P.B.)), the Royal Academy of Engineering (T.D.), the German Federal Ministry of Research and Education (BMBF, 01DR21025A, 01GP2121B, 03ZU1110DD, 13N16486, 01UX2211 (S.R.S.)), the European Research Council (ERC 759370, 101081905 (S.R.S.)), the Foundation for OCD Research (K.J.M.) and the Minnesota Partnership for Biotechnology and Medical Genomics (K.J.M.). We also thank S. Graepel, J. Reindorp and T. Larsson for their help with illustrations, and H. Shao for assistance with background information.
Author information
Authors and Affiliations
Contributions
G.S. initiated and project-managed the creation of this Review, wrote several sections, edited the manuscript, and contributed to the generation of all figures. P.B. edited the manuscript. B.Z.A. developed Fig. 1 and edited the manuscript. S.R.S. wrote the section on macroscale aggregates. C.G. wrote the section on signal processing and AI. T.D. provided the initial requirements based on prior analysis, developed Figs. 3 and 4, and jointly wrote the business/regulatory sections. J.R. developed Fig. 3, jointly wrote the business and regulatory sections, and edited the manuscript. K.J.M. developed Fig. 2 and edited the manuscript.
Corresponding author
Ethics declarations
Competing interests
G.S. previously consulted for CorTec and currently consults for NeuroXess. T.D. is a director and shareholder at Amber Therapeutics, and consults for CorTec. He has previously consulted for Synchron, Inspire and Galvani. J.R. previously served as CEO for CorTec and is a shareholder at CorTec and Neudio. The other authors report no competing interests.
Peer review
Peer review information
Nature Reviews Bioengineering thanks Ramses Alcaide and the other, anonymous, reviewer(s) for their contribution to the peer review of this work.
Additional information
Publisher’s note Springer Nature remains neutral with regard to jurisdictional claims in published maps and institutional affiliations.
Related links
All devices had to be explanted: https://ift.tt/aRuKmhB
BRAIN Initiative: https://ift.tt/HOWDs5R
Covered in the popular media: https://ift.tt/f4CuLl9
Early Feasibility Program: https://ift.tt/GIjQtDm
Epiminder: https://epiminder.com
Humanitarian device exemption: https://ift.tt/UrI6Sjq
InBRAIN: the company’s growth did not meet expectations: https://ift.tt/gtsvzQP
Inspire Medical: https://ift.tt/JWuQwoN
Medical Device Regulation: https://eumdr.com
Medtronic acquires Sapiens: https://ift.tt/Gn40Nrg
NeuroControl Freehand growth: https://ift.tt/gtsvzQP
Neuropace pioneered reimbursement: https://ift.tt/8w7Nfk4
Neuropace Responsive Neurostimulation System: https://ift.tt/95CwTEz
NeuroVista explanted: https://ift.tt/aRuKmhB
NeuroVista explants in popular media: https://ift.tt/f4CuLl9
Nia Therapeutics: https://ift.tt/zR2AJZg
NIH HORNET: https://ift.tt/NEzeBtn
Northstar Neuroscience: https://ift.tt/6HcNlWi
Northstar Neuroscience liquidated: https://ift.tt/JXjTqog
OpenMind Consortium: https://ift.tt/hcdoGBe
Second International BCI Meeting: https://ift.tt/ozyA50W
Second Sight struggles: https://ift.tt/sFkrqnm
Synchron: https://synchron.com
Synchron’s Stentrode: https://ift.tt/HzVpqMa
The company still lost money: https://ift.tt/sFkrqnm
The company was then liquidated: https://ift.tt/JXjTqog
The technology was acquired for US$200m by Medtronic: https://ift.tt/UFxBnjp
Rights and permissions
Springer Nature or its licensor (e.g. a society or other partner) holds exclusive rights to this article under a publishing agreement with the author(s) or other rightsholder(s); author self-archiving of the accepted manuscript version of this article is solely governed by the terms of such publishing agreement and applicable law.
Reprints and permissions
About this article
Cite this article
Schalk, G., Brunner, P., Allison, B.Z. et al. Translation of neurotechnologies. Nat Rev Bioeng (2024). https://ift.tt/hnaV0ym
-
Accepted:
-
Published:
-
DOI: https://ift.tt/hnaV0ym